Evaluation and Testing of Predictions
It is a good practice to test and evaluate your results. In cases where you are using a prediction model together with some incentive for the customer, it is useful to evaluate not only the overall result, but also separate the model, and the incentive. Otherwise, you will not be able to evaluate the real impact of these two separately.
We suggest dividing customers into 4 groups regarding the two parameters:
- High/low probability of reaching the target
- Will/will not be included in the campaign based on predictions. Basically, it is the difference between being and not being in the control group.
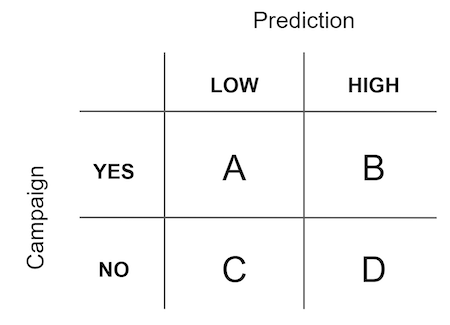
The A and B groups will be included in the campaign while the C and D groups will not.
After the campaign you can calculate the performance of all the groups and calculate the following metrics:
Strength of model
Perf(D) / Perf(C)
Answers how well can the model segment people based on their probability to fulfill the target.
Strength of impression
Perf(A+B) / Perf(C+D)
Answers what can you achieve purely with the impressions without using any prediction model.
Strength of impression on high/low segment
high: Perf(B) / Perf(D)
low: Perf(A) / Perf(C)
Compares wich of the two segments (low/hight) would have higher uplift after a campaign. In other words, which segment is the one where the campaign leads to additional conversions which would not materialize if it was not for the campaign.
Updated over 1 year ago