Autosegments
Autosegmentation uncovers hidden opportunities for marketers by automatically generating high value segments. Using AI to find unique combinations of customer properties and metrics, Autosegments removes the effort needed to analyze data and build hyper-targeted segments manually.
By targeting a more valuable micro-segment, marketers can expect to increase conversions within their campaign, driving 1st time or repeat purchases. Marketers can also tailor the content and recommended products within a campaign, based on the qualitative insights about a segment provided by Autosegments.
How do Autosegments work
Autosegments is powered by Loomi, our AI, to generate potential segments from unique combinations of customer properties and metrics.
Potential segments are presented using a visual, interactive chart that supports further exploration. Marketers can click into each segment for quantitative and qualitative insights, such as a favorite category, average order value, or revenue per visitor, allowing them to pick the best one and create it with a click to use in a personalized campaign.
Segments created via Autosegments feature can be immediately used like any audience segment within Bloomreach Engagement- in Scenarios, Campaigns, Reporting, and more.
Autosegments is currently available only to selected customers
Autosegments is only available to selected Engagement customers, upon approval from the Bloomreach team. If you’re interested in gaining access, please reach out to your Customer Success Manager or Account Manager.
How to create and implement Autosegments
Requirements
1. Create new Autosegment
Go to Analyses > Autosegments and click on + New autosegment.
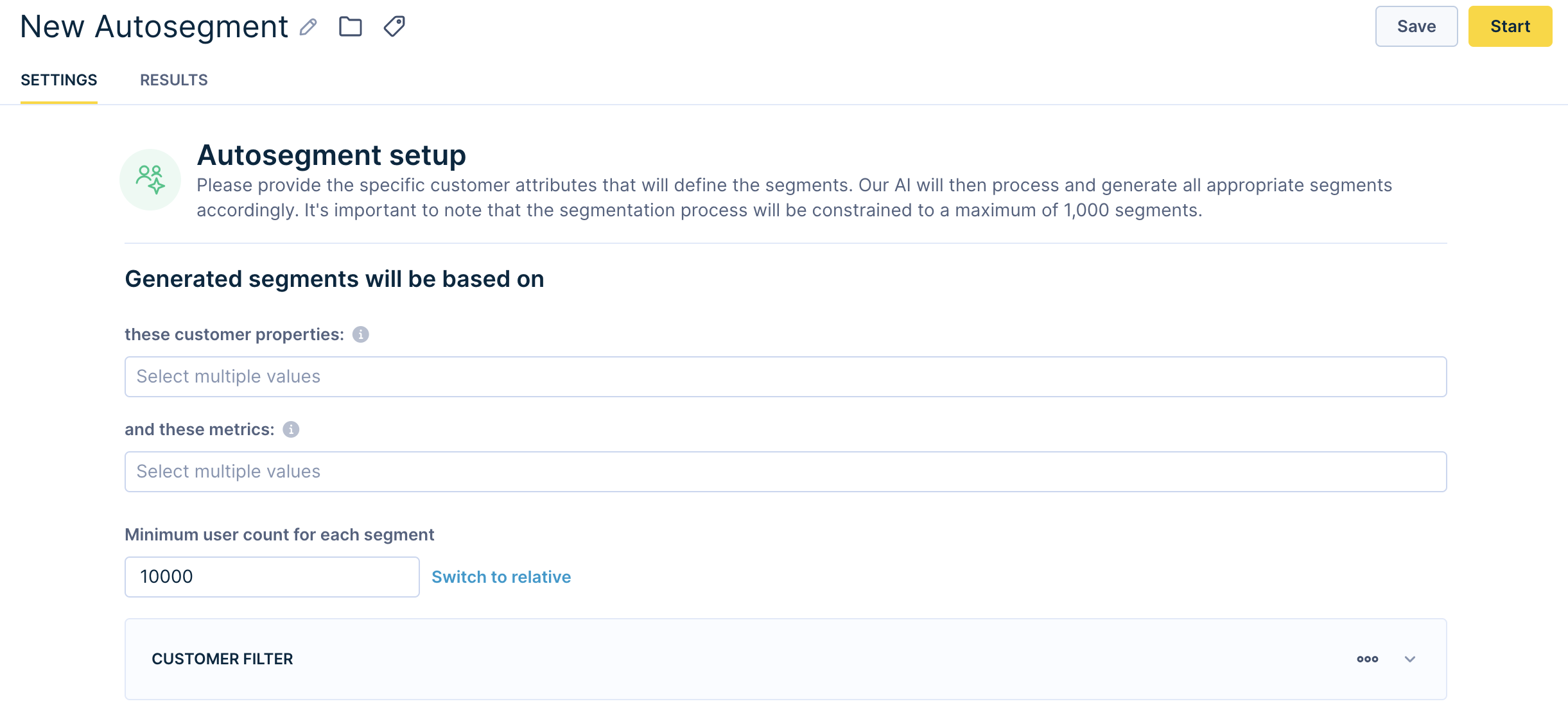
2. Define your values
Select customer properties and metrics on which you wish our AI to base the Autosegments.
You can use a maximum of:
- 20 properties
- 10 metrics
- 5000 segments (if more segments are generated, a random subset is returned)
- last 12 months of data
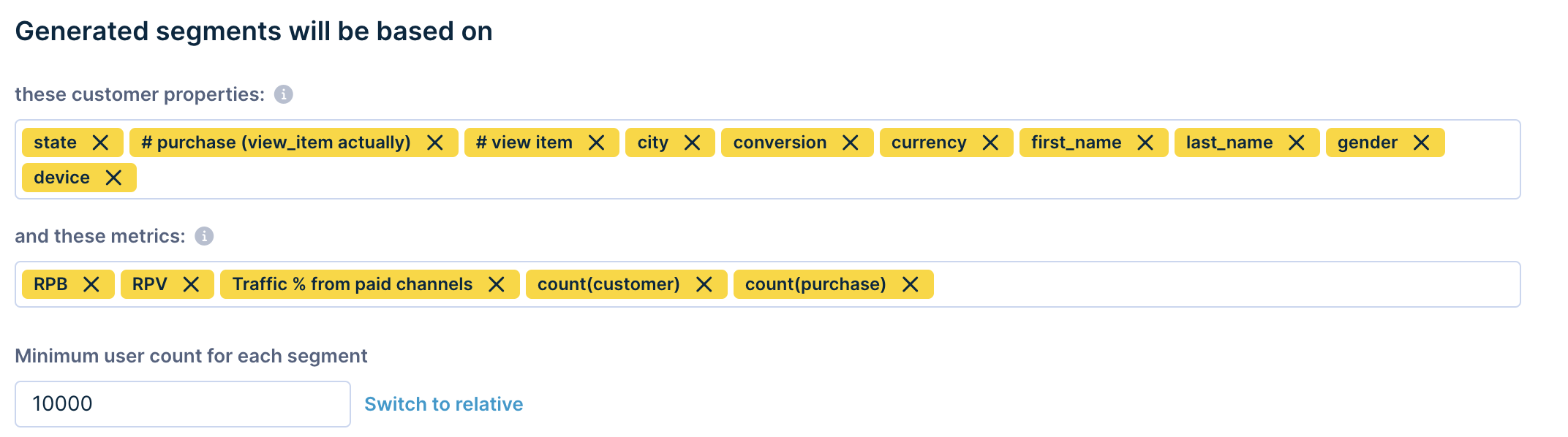
3. Save and start your autosegment
Click on Save and then Start to generate Autosegment. You can see the process in the Results tab. It can take up to 24 hours to generate your Autosegment. The metrics are calculated while running the Autosegment and are not recalculated in real-time when working with Autosegment results.
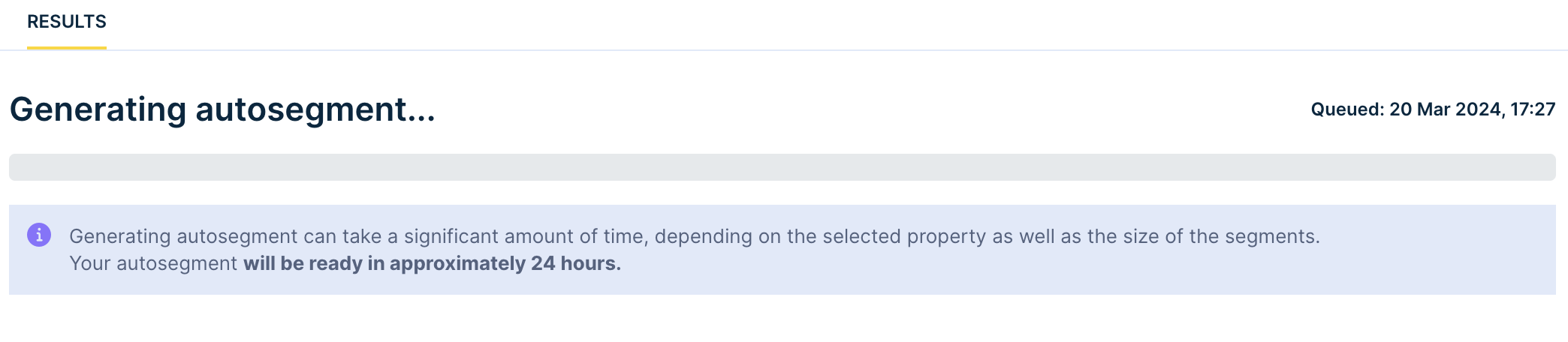
Only segments that meet the defined 'Minimum user count for each segment' are returned as a result.
4. View and analyze the generated Autosegment
Once the Autosegment is generated, you can see the results in the Results tab.
The result is presented as a table with all the segments. The table could be sorted according to the metrics. The segments could also be filtered based on the metrics. The result is also visualized in the form of a diagram.
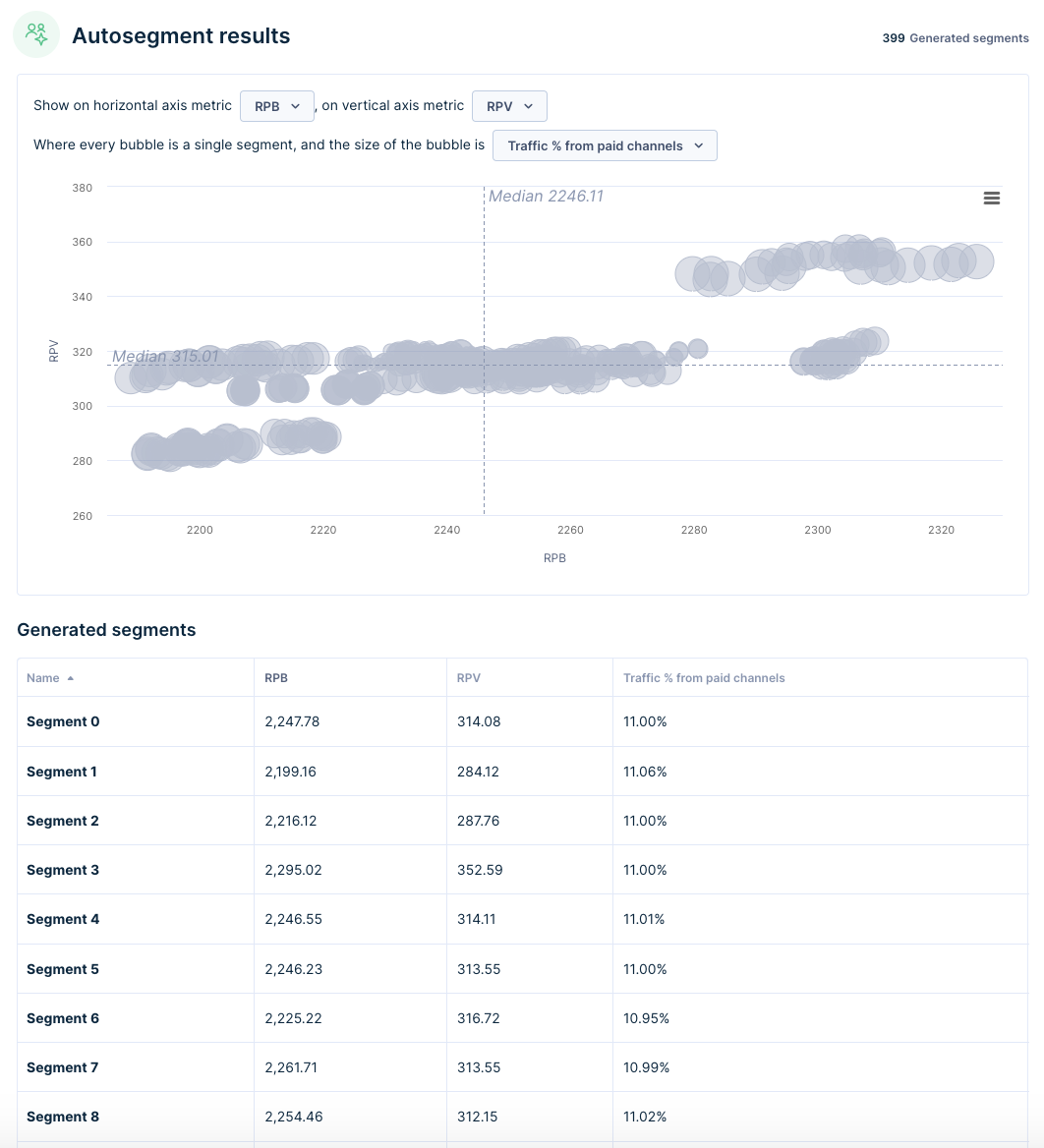
Click on any segment, either in the table or the diagram, and open a modal window with the segment details:
- segment name
- segment description: detail about used properties and values, portion from all customers (100% of customers is the number of customers after applying the customer filter from the setup screen)
- metrics for the specific segment compared to overall project performance
- action button: create segment - this opens a new definition of segmentation with one segment based on the clicked one
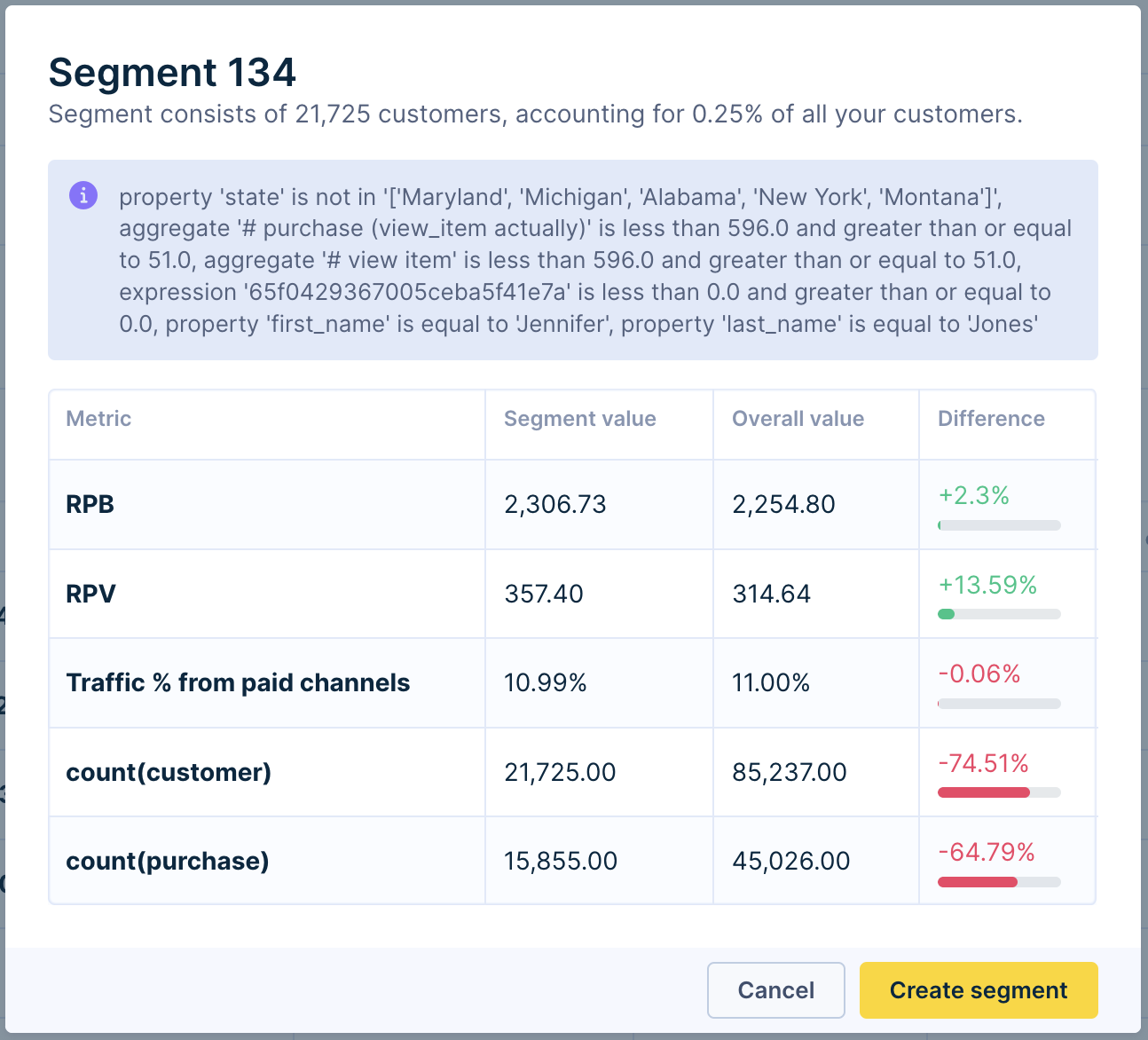
5. Create a segmentation
Choose the segment(s) that you find useful and create a new segmentation. You can then use this segmentation to personalize marketing campaigns or optimize your product offerings.
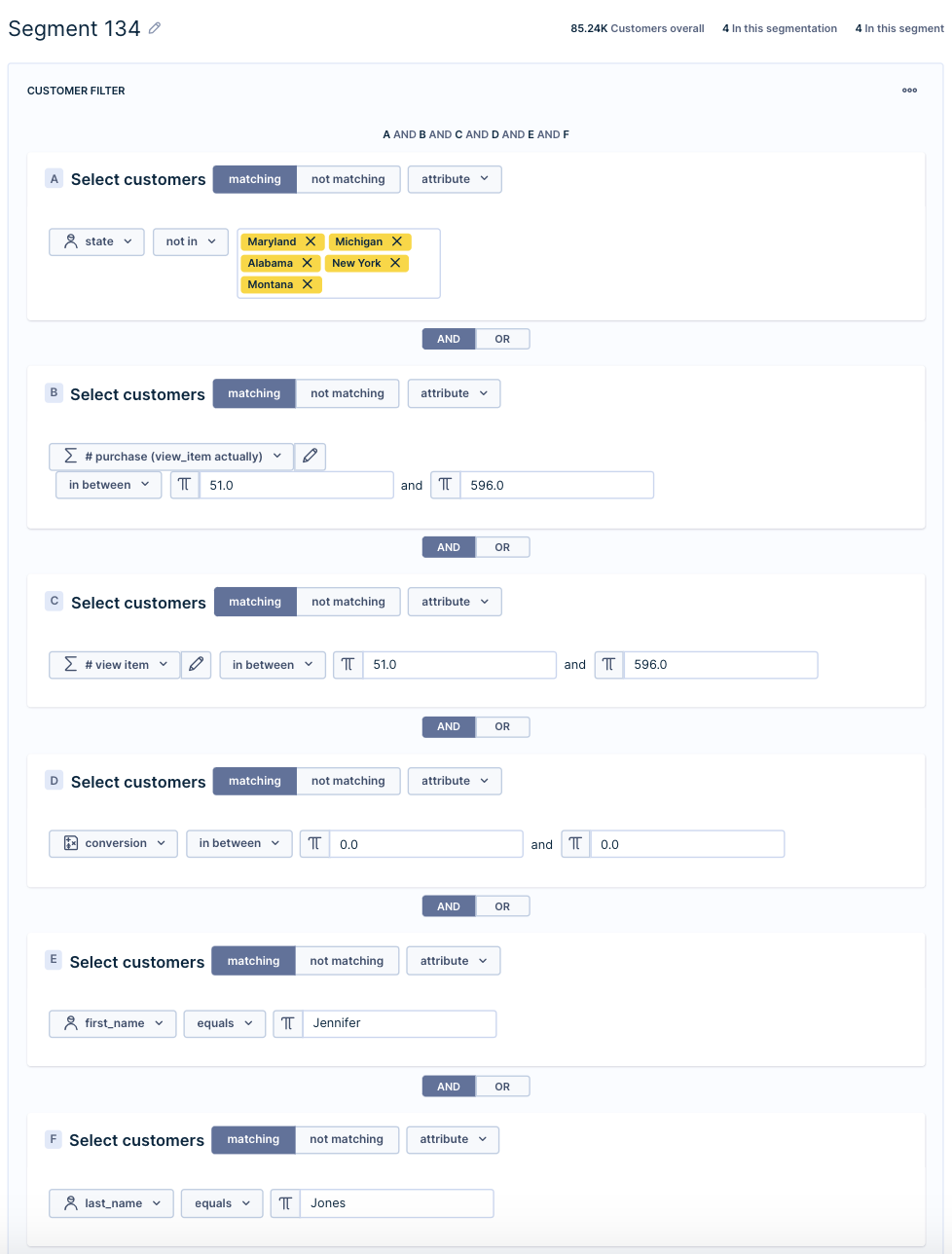
Use case example
The basic scenario is finding a relevant segment that needs to be treated in some particular way. Whether it is to support the segment to increase engagement or not to waste resources on some underperforming segments.
In this example, run the Autosegmetns with the following setup:
- properties: most visited category, gender, interest in flash sales, RFM
- metrics: revenue per visitor (RPV), traffic % from paid channels, revenue per buyer, conversion rate
As a result, you can see many different segments, but when you sort them based on the RPV, you will see some quite underperforming segments. Click on it and see that the 'traffic % from paid channels' is relatively high.
From a business perspective, this means that you invest a lot into this segment, but you are not getting the value back. The action step could be to exclude such segments from retargeting or change the overall retargeting settings to exclude such segments.
Updated 4 days ago